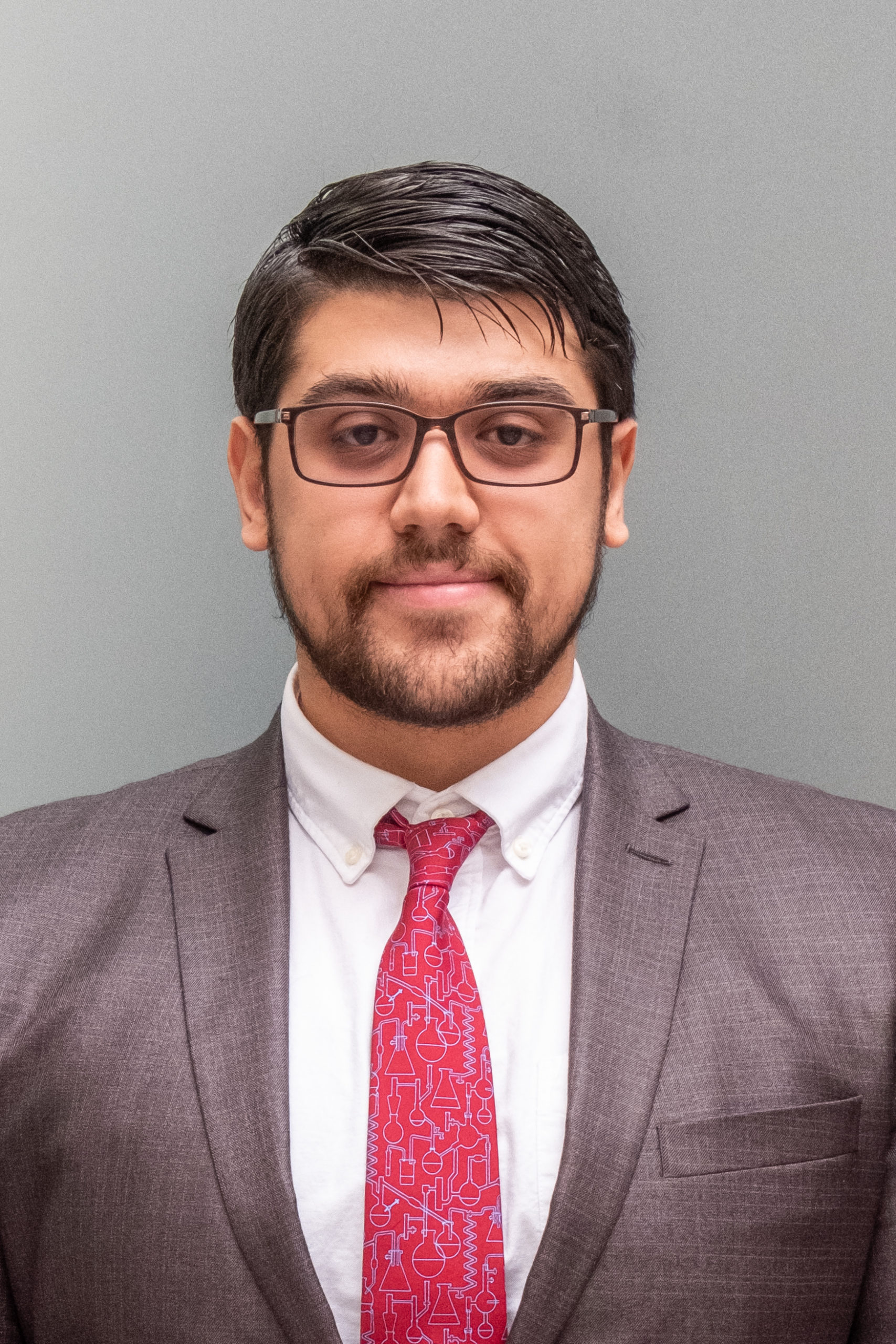
What is clustering, and how is it useful for fragrance safety assessment?
“Clustering,” also called “grouping,” is a process in which scientists organize chemicals into structurally similar groups. Think of how we organize our geopolitical world. First, we organize land masses into distinct areas called “continents.” We then break down those areas into smaller regions defined by more specific features, such as nations and states, down to a single city block populated by similarly designed homes.
(Watch a brief video introducing RIFM’s clustering project.)
This zooming in from a high-level, broad perspective, down to where we can see the individual and comparable features of homes clustered together is similar to how a specific cluster of chemicals is defined by their chemical functionalities in increasing specificity.
Another way of thinking about it would be to consider shopping at a supermarket. First, grocers organize items into aisles defined by their primary characteristics, such as “juices.” Aisles are then further sorted by more specific features, such as types of juice, and then finally by brands of each juice type. This grouping of juice, type, and brand would represent a particular constellation of products, just like a grouping of chemical functionalities would define a specific cluster of chemicals.
If your favorite brand of orange juice is missing from the supermarket, you can easily find a close alternative from the “juices” aisle. Similarly, without sufficient toxicity data on a fragrance material of interest, clustering enables scientists to more efficiently identify structurally similar materials that may serve as read-across analogs (stand-ins for the material).
Clustering saves substantial time and effort—eliminating years in the testing pipeline—and reduces the need for animal testing. It also allows scientists to evaluate several chemicals simultaneously by performing safety assessments on structurally similar materials.
The methodology for clustering has come a long way. Although it was initially based solely on structural similarity scores (such as those provided by the Tanimoto index), researchers have recently started using novel techniques such as artificial intelligence and machine learning to identify the critical properties of the chemical for clustering.
Holger Moustakas, PhD, Senior Scientist, Chemistry, leads the Safety Assessment program’s computational chemistry efforts, including read-across solutions.